Artificial Intelligence (AI) is a concept that corresponds to human intelligence. Since the emergence of this concept in the 1950s, the exploration of human intelligence in AI has gone through different stages. From the early symbolic approach to the establishment of expert systems, it was a top-down design that endowed machines with as much knowledge as possible in advance; over the past two decades, the mainstream paradigm of AI development has gradually shifted to a bottom-up model, endowing machines with the ability to learn, and viewing intelligence as a function that demonstrates adaptability to the environment through learning. Marked by the release of the large language model ChatGPT in 2022, AI neural networks have made milestone progress in human-like learning ability, triggering a global AI boom.
In the 2024 "Government Work Report," the "AI+" initiative was proposed for the first time. This is not only in line with the global trend of AI development but is also closely connected with the overall trend of China's industrial upgrading. The aim is to promote the deep integration of AI technology with various industries. This initiative reflects the government's high regard for AI and can be said to be a policy design and layout to promote China from the "Internet era" to the "AI era." It is an important aspect of developing new quality productive forces.
Advertisement
The new breakthroughs in AI affect economic and social development, and in turn, technological progress is the result of human economic activities. The future development of AI also depends on the evolution of the economic and social environment, including public policy. The China International Capital Corporation Research Institute and the Research Department of China International Capital Corporation jointly wrote this research report, attempting to discuss the productivity characteristics of this round of AI progress and its impact on production relations from an economic perspective, providing a systematic analysis around macro implications, industrial impacts, governance challenges, and other issues.
As a general-purpose technology, the scaling law is a prominent feature of this round of AI progress, meaning that major countries have advantages in a static sense, and pioneers have advantages in a dynamic sense. The United States has a first-mover advantage in the development of large models, and China's large population and market size are conducive to accelerating the catch-up, especially in the application layer, where leading innovations may emerge, injecting new momentum into economic growth. According to our estimates, AI is expected to increase China's GDP in 2035 by 9.8% compared to the baseline scenario, equivalent to an additional annual growth rate of 0.8 percentage points over the next decade. The technological revolution not only promotes productivity but also reshapes production relations. As a "human-like" technology, AI will have a profound impact on digital governance, market competition, social ethics, and international relations. Historical experience shows that technological progress, while increasing economic growth, also widens the income gap. Promoting social security has both a material basis and is an inevitable requirement for sustainable development. China can be well-prepared in AI governance, especially in improving the social security system, balancing efficiency and fairness, and allowing the fruits of scientific and technological development to benefit all people. Against the current backdrop of insufficient total demand, expansionary fiscal policy can boost economic growth and also help China accelerate its catch-up in the AI field.
I. General-Purpose Technology and General Artificial Intelligence
Where do we start in economic analysis of AI? Intuitively, the importance of a technology is related to its impact on the scope of economic and social development. A basic consensus is that AI fits the concept of general-purpose technology (GPT) in economics, with broad application potential, and can play a role in multiple fields and various environments. Technological progress is the source of long-term economic growth, and technological progress across society is often driven by a few key general-purpose technologies. AI has the potential to compare with electricity, computers, the internet, etc., and become a general-purpose technology with significant driving force in the development process of humanity.
In terms of the generality of AI, a related concept is "General Artificial Intelligence" (AGI). The "general" in the field of computers emphasizes the machine's ability to have abilities similar to humans in the fields of reading, speech, images, and other human behaviors. AI large models have initially demonstrated these potentials, such as their core algorithms and technologies can be applied to various different tasks, including data analysis, automated control, etc. But there are many different views on what constitutes AGI, and there is even greater controversy over whether artificial intelligence can reach human intelligence. Optimists and pessimists both exist.
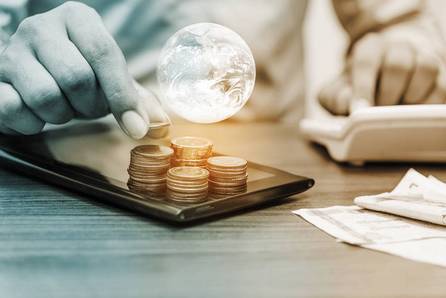
General-purpose technology and general artificial intelligence seem similar, but they are two different concepts. AI is a general-purpose technology, but it may not necessarily achieve AGI. The "general" in the field of economics emphasizes universal applicability (Pervasiveness), but it does not necessarily have to be comparable to human abilities, such as electricity. No one can generate electricity, but human production and life today cannot be separated from electricity. Universal applicability is not achieved in one day. General-purpose technology is a dynamic evolutionary process. New technologies and their applications drive economic and social development, and the latter, in turn, is also a driving force for innovation and application. There is also a synergistic effect between different technological tools, enabling further innovation [5]. AI progress is closely linked to the development of the digital economy and can be said to be the result of the dynamic evolution and empowerment of innovation of digital technology itself as a general-purpose technology.
How to judge the development potential and path of AI as a general-purpose technology? Generally speaking, the development of general-purpose technology follows the "germination-growth-maturity" three-stage S-curve law, and technical feasibility and economic feasibility are two important indicators for judging its dynamic evolution. From the perspective of technical feasibility, the general view is that the technical breakthroughs of this round of AI large models mark that the first inflection point of the S-curve has been crossed. However, in the vast consumer and industrial production scenarios, the penetration rate of AI is still limited and has not shown the widespread application of internet technology. There are economic feasibility issues behind this. The current cost of using AI is high, including the construction of databases, the training of algorithms and talents, and the consumption of computing power. At the same time, the application of large models is still in its infancy, and there is still a large uncertainty in its prospects for generating economic benefits.
The economic feasibility of AI is not static but dynamically evolving. As technology iterates and the scale of application expands, the cost of using AI technology will show a downward trend, and the profitability of business models will gradually emerge. Other industries can use AI to achieve empowerment, transformation, and upgrading, promoting cost reduction and efficiency improvement in their own industries. For example, finance combined with AI can help intelligent investment research and risk prevention, and scientific research combined with AI can achieve automatic literature reading, research plan design, and even control of scientific research equipment and other scientific research assistant functions. In the next few years, the application and promotion of AI large models in various industries will be the carrier for AI to promote economic growth and may have a profound impact.In the long run, the potential impact of AI on the economy and society also depends on where the second inflection point of the S-curve lies. A contentious issue is whether AGI (Artificial General Intelligence) can be achieved and when it will be achieved. Language is the carrier and means of dissemination of knowledge, and some argue that large language models, which simulate intelligence based on language (existing knowledge), naturally find it difficult to produce intelligence that surpasses existing knowledge. How large models trained on historical data can understand and adapt to the dynamic changes in the real world may be a challenge. AI may surpass human capabilities in certain aspects, but it cannot replace human thinking in many fields, especially in innovation.
When discussing the impact of AI as a general-purpose technology on the economy, baseless speculation has no practical significance. We should follow scientific methodology and build rational analysis on a logical framework. Whether it is the development of AI itself or its impact on the economy, a key term in the analysis logic is economies of scale.
II. Scale Law and Economies of Scale Effects
An important concept in economics is the economies of scale effect, which means that an increase in production scale leads to a decrease in unit costs and an increase in efficiency. There is a similar concept in the current round of AI technological progress, which is the scale law. This refers to the systematic improvement in performance as the model size increases. With the support of algorithm optimization, data increase, and computing power growth, large language models can include hundreds of billions to trillions of parameters, helping machines learn complex patterns in language data. The scale law describes technical feasibility, while the economies of scale effect concerns economic feasibility. The two are interconnected, and to some extent, it can be said that the former is the foundation of the latter, and the latter is the carrier of AI's impact on economic operations, which in turn also affects technological evolution.
Looking at future development, a key question is whether there is a limit to the efficiency improvement brought by scale increase, and where the boundary is? From the perspective of model technology, there are two schools of thought. The optimistic school believes that after crossing the first inflection point of the S-curve, the development of AI is far from reaching the limit of the scale law. Following the direction indicated by the scale law, combined with more efficient algorithm architectures, higher-performance computing power chips, and the application of more data, we can expect continuous breakthroughs in AI's technical performance in the coming years. The cautious school believes that the marginal output of increased computing power, data, and parameters has already shown signs of decline, and the use of data volume from stock to reliance on increments also faces limitations.
The application of large models is not only a technical issue. Even if the marginal output of increased data scale (model prediction accuracy) decreases, if the benefits generated by its application are greater than the input costs, economic feasibility still holds. Economies of scale effects are key factors in AI applications and industrial development. There are two aspects of economies of scale in economics: internal economies of scale and external economies of scale. The former refers to the efficiency improvement (unit cost reduction) achieved by a single company by increasing production and business scale, while the latter refers to the efficiency improvement achieved by companies in the industry chain through collaboration or sharing infrastructure and public services. In the digital economy era, the efficiency improvement within a company may not necessarily be reflected in the increase of a single product's quantity, but may also be achieved through the expansion of business scope and the increase of product types, that is, the scope economy effect.
Specifically, the internal economies of scale effect of AI is reflected in the efficiency improvement brought by the increase of operational scale for a single company with a large model. The scale law at the technical level implies a threshold requirement at the economic level. When computing power, parameters, and data volume reach a certain scale, the accuracy and capabilities of the model experience a leapfrog improvement, which is the so-called emergence. This nonlinear effect brings a certain threshold requirement for the development of AI large models in resource investment, combined with the scope economy effect at the application level, leading large technology companies to be more capable of achieving internal economies of scale.
The external economies of scale of AI can be reflected in three aspects. First, driven by large language models, the market has given unprecedented attention to the AI field, and more and more capital is invested in the research and development of new algorithm architectures, databases, and computing infrastructure construction. This helps to reduce the average cost of algorithms, data, and computing power in the entire market, which is beneficial to all market entities. The lack of computing power among Chinese companies may limit the performance of their internal economies of scale, but this can be compensated to some extent through external economies of scale such as infrastructure and public services (sharing).
Secondly, external economies of scale are reflected in the interaction and mutual empowerment between model developers and users. For example, open-source large models can attract developers from universities, companies, and individuals to call, who discover problems during use and provide feedback and suggestions for modification through online platforms, thus forming a division of labor and collaboration network, accelerating technological iteration and progress.
Finally, as AI technology spreads from technology companies to other industries, related companies can integrate their own business with AI to form industrial chains and ecosystems, achieving division of labor and collaboration within and between industries, bringing external economies of scale effects. As large models iterate and improve, industries such as scientific research, medical care, and finance will be able to develop customized small models for use in daily business activities, further enriching the technical ecosystem. Small models from different industries can learn from each other's knowledge and experience, creating new public data to feed back into technology research and development, achieving scope economy effects.Human history demonstrates that the economies of scale brought about by technological progress are not infinite; eventually, increasing marginal returns turn into diminishing marginal returns. We need to think beyond the technical aspects when considering the economies of scale for AI, as technological advancements are endogenous. Even if the second inflection point is far from us, the expansion of scale itself may face constraints. Socioeconomic resources are finite, and human needs are diverse, making it unlikely to invest all resources in a single technology or industry.
From a socioeconomic perspective, a macro constraint is related to climate change, with energy consumption and carbon emissions becoming one of the public's focal points in this round of AI advancements. AI's lifecycle requires electricity consumption during both the training and inference phases, increasing carbon emissions. On the other hand, AI can aid in energy transition and reduce carbon emissions, such as helping to develop new clean energy technology materials and optimizing the operation of solar and wind power generation farms. Which of these forces is more significant? The history of economic development shows that technological progress helps to reduce energy consumption per unit, but the pursuit of a better life leads to economic growth and an increase in total energy consumption.
Addressing the impact of AI advancements on carbon emissions requires accelerating the green transition and promoting the replacement of fossil fuels with green energy. Green energy has strong manufacturing characteristics and economies of scale, and China, as a major manufacturing country and large market, can make significant contributions to the global green transition. However, the development of China's green industry has already led to protectionist pressures, reflecting competition between countries on economic and geopolitical levels.
III. The Great Convergence and the Great Divergence
Technological progress has a significant impact on the economic competitiveness and development gaps between countries or regions. During the Industrial Revolution, Western countries (such as the UK, Germany, and the US) experienced rapid economic growth, while Eastern countries, represented by China, gradually fell behind, leading to significant changes in the global economic and political landscape. This phenomenon is known in economic history as "the Great Divergence." After World War II, a few economies (mainly in East Asia) successfully caught up with developed economies during the process of industrialization. Since China's reform and opening up, its rapid economic growth has significantly narrowed the gap with developed countries, which are considered examples of "the Great Convergence." Today, with rapid technological progress, some worry that the world is experiencing a second Great Divergence due to the concentration of technological innovation and industrialization in a few countries, widening the gap between advanced technology countries and traditional manufacturing countries.
Will this round of AI advancements lead to divergence or convergence? Two schools of thought in economics help us understand this issue, with the key being the understanding of technological progress and economies of scale. According to neoclassical growth theory, technological progress is exogenous, and the marginal return on capital decreases, bringing latecomer advantages to developing countries. The marginal return on capital in developing countries is higher than in developed countries, capital flows from high-income countries to low-income countries, and learning and imitation lead to the diffusion of technology, making the economic growth of the latter faster than the former, and the gap in per capita income levels tends to converge.
Endogenous growth theory emphasizes that technological progress is endogenous, and economies of scale are an important force. Compared with the agricultural economy, the industrial economy has economies of scale, allowing countries that industrialize first to invest more resources in R&D and innovation, giving them a first-mover advantage in cutting-edge technological progress, leading to non-convergence in income levels across countries.
Another implication of the two schools' differences is that, under the same conditions, the endogenous growth model implies that large countries grow faster than small countries, and large countries are richer than small countries, while neoclassical growth theory believes that economic growth rate is unrelated to scale. Following the logic that technological progress is endogenous, on the supply side, large countries have more resources to invest in R&D and innovation, and on the demand side, a large market means greater profit space for innovation, while the spillover effect of technological progress is stronger in large countries.
What are the implications of the scale law of AI large models for the scale effects in the economic sense, both statically (large countries have advantages over small countries) and dynamically (first movers have advantages)? The emergence of AI large models (non-linear characteristics) or input threshold requirements give large countries a scale advantage in the development process of AI. Large countries have more people and enterprises, which helps to share high fixed (training) costs and break through the scale threshold of AI technology more quickly. At the same time, large countries have more users and scenarios for AI, bringing a large and rich local data set, as well as a larger and more diverse application market, thus achieving a stronger learning-by-doing effect. Large countries are also more likely to build infrastructure conducive to the application of AI, helping the diffusion of products and technology.
The scale effect means that this round of AI advancements is more favorable to the two largest economies in the world, China and the United States. The United States has a larger economic volume than China, but China has a larger population than the United States. Capital and labor are partially substitutable but not completely substitutable, so from a scale perspective, China and the United States each have their advantages and disadvantages. However, in terms of this round of AI advancements, the United States has a first-mover advantage. In the traditional deep learning era, there was a slight gap between China and the United States in AI development, but they were basically in a state of parallel development or各有千秋. In the past few years, with large models as a symbol of AI advancements, the United States has maintained a relative lead, and there are signs of the gap widening. How to view future development? We can discuss it from the computational layer, model layer, and application layer respectively.Even in terms of the force layer, there are signs that the traditional Moore's Law is facing its limits, and the chip manufacturing process is entering a stage of diminishing returns to scale. This implies that the importance of the first-mover advantage of developed countries is decreasing, providing space for China to catch up with advanced levels. At the same time, new technological routes such as new computing architectures and non-silicon-based semiconductors are still in the early stages of research and development, and there is a possibility for China to accelerate its catch-up.
From the perspective of the model layer, after the first inflection point of the S-curve, large models enter a stage of increasing returns to scale, which means the United States has a first-mover advantage. The extent of the United States' first-mover advantage partly depends on how far the second inflection point of large models on the technical level is, or where the technological limits faced by the leader itself are, which currently has a large degree of uncertainty. At the same time, the United States is trying to strengthen its first-mover advantage by introducing relevant measures to ensure the free flow of AI-related talent and infrastructure. Combining the universality of AI large models and the R&D investment threshold implied by the scale law, the latecomer disadvantage may lead to insufficient spontaneous catch-up incentives for Chinese companies.
In terms of applications, many countries, including the United States, are exploring the application scenarios of large models, and there are still few mature successful cases, so the first-mover advantage of the United States is not obvious. In the exploration of the application layer, China's rich application scenarios and diverse market scale advantages in potential demand are obvious, which not only helps to accelerate the catch-up but may also give birth to some innovative and leading innovations.
As a general-purpose technology, AI enhances the efficiency of the entire economy through external economies of scale at the application layer, which is reflected in the supply and demand sides, upstream and downstream linkages, mutual empowerment, and promotion of innovation. AI large models use big data for simulation and prediction, which is conducive to the imitation ability of latecomers. It not only empowers the traditional imitation of "knowing why" but also increases the new imitation ability of "only knowing what", all of which help catch-up innovation. The implications of enhanced imitation ability for innovation that relies on originality are not clear. On the one hand, imitation erodes the monopoly profits of leading innovators, suppressing the willingness to innovate. On the other hand, imitation products intensify market competition, and the resulting decline in the average profit rate of the industry prompts companies to strengthen leading innovation to maintain excess profits. Which of these two forces is stronger, and the scale effect is also a key factor. The profit space provided by a large market motivates innovation investment in a competitive environment.
Looking at the main driving force of technological progress, before the first inflection point of the S-curve, the development of AI technology is more reflected in supply-side driven innovation, and large language models are a prominent result. After the first inflection point is crossed, AI industrialization is key, which is the carrier of technological progress promoting economic growth, and the commercial profits brought by industrialization also promote the iterative innovation of AI technology. Therefore, at the current stage, the application on the demand side is key. From the perspective of application, China has a scale advantage. In terms of serving ordinary users, China has a large population, and the potential demand for AI industrialization is vast. In terms of serving enterprise users, China's industrial system is complete, which can provide a rich application scenario for industrial AI. To make good use of China's scale advantage, public policy needs to create a macro environment conducive to innovation, including strong consumer demand, a prosperous capital market, effective digital economy governance mechanisms, and public infrastructure.
China's scale advantage is conducive to playing the role of AI progress in promoting innovation within the economic system. The large market for single products has an incentive effect on imitators, and diversified demand promotes leading innovation. Only a few large economies have a large enough market to accommodate multiple sub-tracks while each sub-track has a large enough volume to achieve economies of scale, thus balancing catch-up and leading innovation.
To make good use of China's scale advantage, a key issue is how to promote resource investment in innovation. For catch-up innovation, its technical path is relatively clear, and the advantages of large enterprises in accumulating innovation elements (intellectual property rights, human capital, R&D investment, etc.) should be utilized, with banks providing long-term and stable financial support. Leading innovation usually has no mature technical path for reference and is more dependent on the innovation trial and error of numerous small and medium-sized enterprises. The capital market can more effectively play a role in screening innovative technical routes and business models. China's scale advantage is particularly prominent in the manufacturing industry, and a new growth point is humanoid robots.
IV. Humanoid Robots and Machine Replacement of Humans
Technological progress affects the economy in two aspects: replacing labor and empowering labor. On the one hand, technological progress brings new production tools such as automated machinery and equipment, allowing capital to partially replace labor. On the other hand, technological progress helps workers complete the same work tasks in less time, improving labor productivity. AI also has two effects of replacing and empowering labor, but the mode and intensity are different from past technological progress. Past technological progress replaced labor more in labor-intensive fields such as agriculture and low-end manufacturing. AI progress, especially large language models and other technologies, has shown the ability to replace some mental labor, such as translation.
Replacing physical labor and replacing mental labor are not mutually exclusive. An important potential of this round of AI technological progress is the combination of the two, which is reflected in the development of embodied intelligence. Embodied AI refers to an intelligent agent having a body and obtaining intelligence through the interaction of the body with the environment. This interaction includes using sensors to perceive the environment and using actuators to affect the environment. The intelligent agent learns and adapts through physical interaction with the external world. For example, allowing robots to learn how to perform tasks such as walking and grasping objects without explicit instructions, through exploration and experimentation.Embodied intelligence can be said to be a deep integration of artificial intelligence and robotics, with an important application being humanoid robots. Humanoid robots refer to robots that have an appearance and functions similar to humans, and can perform tasks that humans can complete to a certain extent, such as walking, operating tools, and communicating. The breakthrough in AI in this round has enhanced the technical feasibility of humanoid robots, with natural language processing, facial recognition, and expression simulation contributing to more natural communication between machines and humans. Humanoid robots can replace or assist human labor in various fields, such as manufacturing, medical care, and service industries, thereby improving production efficiency and service quality. The popularization of humanoid robots will encourage more labor to shift from physical labor to creative and managerial work, which is not only conducive to increasing the added value of work but also to improving working conditions and reducing work-related accidents.
The promotion and popularization of humanoid robots are not only issues of technical feasibility but also economic feasibility. The manufacturing industry that produces robots has economies of scale, and breakthroughs in technical barriers rely on investment in research and development and innovation. A large market is a key factor in promoting innovation momentum. With design optimization brought about by innovation and large-scale production, the production costs of humanoid robots are expected to continue to decline. The combination of economies of scale in manufacturing and digital technology gives China a unique advantage in the development of humanoid robots.
China already has the world's largest industrial robot application market, with industrial robot installations accounting for more than 50% of the global share in 2022[18]. Humanoid robots are expected to bring new opportunities for industrial development. On the supply side, the production of humanoid robots is inseparable from manufacturing, and China's manufacturing industry has a complete range of industries and a complete industrial system. On the demand side, China's larger segmented market provides a broad testing and deployment environment for the application of humanoid robots, such as factory manufacturing, elderly care, and dangerous rescue departments, which can accumulate a large amount of data, helping AI technology to iterate and optimize. At the current stage in China, the development of humanoid robots is not limited to home use but also shows greater application potential in industrial fields, including manufacturing and mining. The development of AI may also bring new technological paths, reducing the knowledge premium of traditional developed countries such as Japan and the United States in robot manufacturers. China's development of the humanoid robot industry, like the development of the new energy vehicle industry, has the potential to overtake.
Of course, the development of humanoid robots will be a global phenomenon with universal significance, and there are a series of macro and structural implications worth exploring. The replacement of humans by machines naturally leads to debates about the number of people. On the one hand, humanoid robots, as an advanced technological solution, provide new possibilities for addressing labor shortages caused by population aging. On the other hand, the replacement of humans by machines raises concerns about large-scale unemployment, especially the prospect of the popularization of humanoid robots, making the term "technological unemployment" (unemployment caused by technological progress) a topic of public concern.
How should we understand this issue? The concept of "Baumol's disease" in economics[19] provides a useful analytical framework. Its logic is that resources (including labor) shift from sectors with rapid efficiency improvement (supply exceeds demand) to sectors with slow improvement (supply does not meet demand). The speed of labor productivity improvement brought about by technological progress varies between different sectors, and there are always industries and fields with relatively slow labor productivity growth. At the same time, human desires are a psychological concept and are infinite in some aspects, with some demands always unmet and requiring resource investment.
In the 1960s when Baumol published his articles, manufacturing efficiency improved rapidly, while the service industry was slow, so resources shifted from manufacturing to the service industry. The proportion of the service industry in developed countries increased, and the proportion of manufacturing decreased. However, if machines replace humans more in the service industry now, it means that the service industry's efficiency improvement is rapid, and the supply of manufacturing robots may not keep up with demand. Labor needs to shift from the service industry with rapid efficiency improvement (machines replacing humans) to the manufacturing industry that produces robots and those service industries with slow efficiency improvement. Although it is difficult to accurately predict the changes in future employment structures, the general logic should be that the surplus labor generated by industries with rapid technological progress will eventually be absorbed by industries with slow technological progress and supply that cannot meet demand. Of course, this adjustment may be a painful process for some industries and groups, requiring support and assistance from public policies.
In addition, the rise in the proportion of global manufacturing also means the increased importance of physical resources, such as the increased demand for materials like steel, copper, and aluminum, leading to relative price adjustments of goods. This may also increase energy consumption, increase carbon emissions and pollution issues, and increase the pressure of global green transformation.
V. Ethics and Governance
Beyond the economic level, the impact of humanoid robots on society, culture, and ethics is also worth paying attention to. Humanoid robots can be used in multiple fields such as education, medical care, and household services, and may change human perceptions of these fields and social roles. Social ethics and governance mechanisms are important issues concerning the development of all AI technologies, including humanoid robots. Currently, in the discussion of AI ethics and governance, there are three topics worth paying special attention to: first, the governance of data elements; second, the market power of large companies; and third, broader social equity and security issues.
Firstly, data is a basic production element of the AI industry, and data governance is a key part of AI-related governance mechanisms. Although some people compare data to oil in the digital economy era, from an economic perspective, data has an essential difference from non-renewable production elements like oil due to its non-competitive characteristics - that is, it can be reused by different subjects and the marginal cost approaches zero. Non-competitiveness brings externalities, and the production and use of data not only involve directly related individuals or organizations but may also have an impact on other members of society, including positive and negative impacts.Positive externalities stem from the network effects of data, meaning the value of data often increases with the growth in volume and diversity. The scale law of AI implies that only data of a certain scale can realize its value in the training of large models. Multimodal models further demand diversity in data, which comes from various sources and takes on different forms and types, such as text, images, videos, etc.
Negative externalities manifest as the generation and use of data potentially leading to privacy and security issues. The collection, storage, use, and transmission of personal data, while creating economic value, also pose risks of privacy leaks and data misuse. Data can be easily copied and disseminated, which, while beneficial for the spread of knowledge, may also lead to disputes over intellectual property rights. There may be information asymmetry between data generators and users, making the contradictions between the aforementioned positive and negative externalities more pronounced. This poses challenges for data to leverage economies of scale, either because the scale is insufficient to fully exploit the value of data, or because large-scale use can easily lead to abuse issues.
Externalities imply that data should be regarded as a quasi-public good, requiring policy-level intervention and regulation. It is necessary to promote the open sharing of data to leverage its economies of scale while also protecting citizens' privacy and data security. The production and use of data require corresponding governance and regulatory mechanisms. At present, a key focus is on the circulation process. Data can only incentivize production when its value is fully realized in the circulation process.
Globally, there are two main ways for data circulation. China mainly conducts transactions through local exchanges, while developed countries like the United States primarily engage in over-the-counter transactions through data brokers. In reality, although China's total data volume ranks second in the world, its data market size in 2021 was less than 25% of that of the United States[20]. Given the difficulty in defining property rights of data elements, the dispersion of rights holders, and the complexity of the pricing process, overemphasizing the standardization of transactions through data rights confirmation may increase the transaction costs of data circulation, and macroscopically, it may not achieve the goal of scaled circulation.
Solving these issues requires exploring different methods and paths in practice, and comprehensively managing from the technical, managerial, and policy levels. At present, it is particularly important to promote the public disclosure of non-sensitive data by governments and public sectors, and to encourage enterprises to innovate in data production and use. Possible measures include strengthening the market competition constraints faced by state-owned enterprises to enhance their focus on data collection and use; providing economic incentives to large manufacturing enterprises, especially private ones, to support their database construction and AI transformation; and in precision manufacturing fields where "bottleneck" issues such as chips are prominent, focusing on creating a catch-up innovation model led by large enterprises, facilitating the collection of scarce data through vertical integration.
The second aspect of AI governance is that the development of large models may increase concerns about the market power of large enterprises. Monopoly was once a focal point in the governance of digital economy platforms, but since entering the era of AI large models, discussions on monopoly seem to be less frequent. An important context is that AI large models, as disruptive innovations, are having a significant impact on the monopolistic power of platform enterprises in the digital economy era. For example, in the search engine market, although Google's global market share remained as high as 90% as of April 2024[21], there have been many speculations in the market about which AI application will disrupt Google Search[22]. Additionally, the generalization ability of AI large models in various fields is still constrained by technical conditions, and the competition among various large model startups is fierce, both in the basic large model field and in vertical application fields.
Although monopoly is not the main risk currently faced by the development of AI large models, will this field form new market forces that are detrimental to competition in the future? The scale law from a technical perspective tells us that with the continuous advancement of technical capabilities, multimodal AI large models may "winner takes all," replacing other models with average performance. The scale effect from an economic perspective tells us that AI large models have network effects, with high upfront development costs but low marginal usage costs, which may form a situation favorable to a few large enterprises.
When discussing market power and its governance, there are two main approaches: structuralism and behavioralism. The former believes that industrial organization structure determines corporate behavior and advocates breaking up monopolistic structures through antitrust measures. The latter believes that structure is the result of behavior, and the focus of antitrust should be on anti-competitive monopolistic behaviors rather than industrial organization structure[23]. Which approach is more suitable for the era of large models? Whether it is conducive to innovation is an important criterion. AI large models, due to their scale effects, are more conducive to large enterprises increasing their market share, but the key is whether the relevant enterprises have anti-competitive behaviors that hinder the free flow of data, talent, and other elements.
At present, China's AI field is facing dual challenges in innovation: it must catch up with the level of world-class large models and also look far ahead to achieve leading innovation. Large enterprises play a more important role in catch-up innovation, while small enterprises are the main force in leading innovation. Structuralist thinking may suppress the innovation of large technology enterprises, while behavioralist thinking can better balance the relationship between large and small enterprise innovation. Instead of focusing on the scale of enterprises, it is better to focus on the competitiveness of the market, such as preventing monopolistic behaviors of enterprises and promoting the free flow of data, computing power, talent, and other elements.
The third aspect of AI governance is the ethical and security issues at the geopolitical level. The application of AI technology in high-end manufacturing may lead to new ethical and security issues. The AI industry is technology-intensive and closely related to the economy and society, which is highly valued by all countries. In the past two years, more than 60 countries around the world have successively introduced AI development strategies[24], but the scale effect and the first-mover advantage associated with it may put major countries, especially the United States, in a more favorable position. The development of AI will bring new challenges and transformation pressures to the international governance mechanism.Six, Efficiency and Fairness
Artificial intelligence, as a general-purpose technology, while enhancing production efficiency, may lead to changes in the economic and employment structure, widen the gap in income distribution, and bring new challenges to balancing efficiency and fairness at the social level. At the aggregate level, it is manifested as a relative insufficiency of demand compared to supply, which has important implications for macroeconomic policy. Economies of scale are an important carrier connecting the efficiency and fairness at the micro level with the balance of total demand and total supply at the macro level. Economies of scale amplify the efficiency-enhancing effects of technological progress, but they may also widen income disparities and exacerbate the problem of insufficient total demand.
From the supply side, the integration of AI with various industries is expected to increase the potential growth rate of the economy. Unlike machine automation that requires pre-programming, this round of technological progress has endowed AI with stronger generality, allowing it to participate universally across scenarios in human work task processes, enhancing total factor productivity (TFP) through both substitution and empowerment of humans. As AI capabilities improve and costs decrease, the scale of industries integrating with AI will gradually expand, and the economic enhancement effects will become more significant. We estimate that by 2035, AI could increase China's GDP by 9.8% compared to the baseline scenario, equivalent to an additional annual growth rate of 0.8 percentage points over the next decade. The penetration and application diffusion of AI technology require time, which means that the enhancement of production efficiency in economic growth will exhibit a pattern of being low at first and then increasing.
The enhancement of supply capacity is not something that can be achieved overnight; the penetration of technology and commercial application take time. Although AI changing our lives has become a popular topic, many businesses are still looking for viable business models. One aspect is the support of initial investment; to achieve broadly significant efficiency improvements, large-scale investment is needed in software, hardware, and robot production. The premise for AI to significantly enhance economic efficiency at the macro level is to first observe a substantial increase in capital investment, which is also a perspective for us to observe new economic growth points.
In the long run, compared to the enhancement on the supply side, the widespread use of AI technology has a relatively smaller effect on promoting total demand, leading to a macro pattern of supply exceeding demand. This is because technological progress intensifies the gap in income distribution, and the marginal propensity to consume of high-income individuals is usually lower than that of low-income individuals. The expansion of income inequality reduces the overall consumption propensity of society, suppressing the growth of final demand (consumption).
Compared to past technological progress, AI, as a "human-like" technology, may be more disadvantageous to workers in terms of income distribution. An IMF report suggests that about 40% of global jobs will be exposed to AI, with this proportion reaching as high as 60% in developed economies. Under the influence of market mechanisms, the possibility of large-scale unemployment is small, but the cost is that wage growth is slower relative to the return on capital. AI technology itself is a product of R&D, and high returns mainly belong to innovators and venture capitalists. At the same time, the commercial application of AI technology, especially the popularization of humanoid robots, requires a large amount of investment. Capital deepening means a decrease in the share of labor income in GDP. Moreover, this round of AI progress may have a greater impact on white-collar workers in the middle of the social income spectrum, exacerbating the polarization of income distribution among workers.
With the promotion of AI applications, a situation may arise where the benefits of technological progress are privatized, and the costs of addressing the problems brought by its impact are borne by the entire society. The most prominent manifestation of this cost at the macro level is insufficient total demand. What are the implications of insufficient total demand for public policy? Fiscal and monetary policies are the two levers for adjusting total demand. Monetary policy is a counter-cyclical adjustment tool for the total amount and has limited effects on structural issues such as income distribution, and may even have a counter-effect. Fiscal policy can both make counter-cyclical adjustments to the total amount and be an effective tool for adjusting structures and reducing income inequality. In response to the inequality issues brought about by AI progress, fiscal policy should also play a key role in promoting the benefits of technological progress to benefit all people.
In terms of fiscal expenditure, Universal Basic Income (UBI) has become a hot topic internationally in recent years. Its core is to provide an unconditional and universal income guarantee, allowing the entire population to enjoy the benefits brought by technological progress. Although there are many controversies, the proposal of related concepts reflects the urgency of enhancing social security to balance technological progress and social welfare in the digital economy era.
Looking back at the history of developed countries, technological progress and economic growth have led to the establishment and gradual improvement of the social security system. The initial form of the modern social security system emerged after the Industrial Revolution, such as the workers' insurance system implemented in Germany at the end of the 19th century. The second technological revolution, characterized by the widespread application of electricity and the internal combustion engine, accelerated the process of industrialization, during which the social security system further developed, including the establishment of pension systems and the implementation of medical insurance. Since the third technological revolution characterized by the popularization of computers and the internet, the scope of social security has further expanded, such as unemployment insurance, family allowances, and long-term care insurance.
Since the reform and opening up, China's social security system has evolved from an initial single-tier protection system to a multi-level, widely covered social security system. Although the social security system has achieved a transformation from partial coverage to universal coverage for all, a prominent issue remains the lack of fairness. Social insurance programs such as pension, medical care, and unemployment insurance, as well as social assistance centered on the minimum living standard guarantee system, are still organized by urban and rural areas or groups, with significant differences in the level of protection and social security benefits among different groups. In particular, the protective functions of some programs are insufficient, such as rural residents' pensions, which can only play a supplementary role, and the minimum standard of living guarantee in some economically underdeveloped areas still needs to be further improved.These issues and challenges require resolution through further reforms, and technological advancements, especially the economic supply enhancement brought about by the current round of AI development, provide a foundation for improving the social security system. Economic growth and wealth increase driven by technological progress make society more generous and capable of helping vulnerable groups, strengthening the safety net for low-income populations, particularly enhancing social security for migrant workers and rural residents, which contributes to promoting common prosperity. At the same time, the application of new technologies such as AI and big data analysis also offers new possibilities for the distribution, management, and supervision of social welfare, helping to improve the efficiency and transparency of social security.
How can the fiscal expenditures resulting from improved social security be compensated? Currently, the lack of demand is due to both structural issues and the downturn in the economic cycle. Expansionary fiscal policy can combine stable growth with structural adjustment, promoting the unity of efficiency and equity. In other words, the fiscal sector can raise funds to improve social security and public services by increasing the issuance of government bonds rather than taxes. Government debt stimulates demand, demand realizes supply potential, promotes innovation and technological progress, and enhances future growth and debt repayment capacity.
It should be noted that the development and industrial application of AI technology are still in their early stages and are not the cause of the current insufficient total demand. What we need to pay more attention to now is the endogeneity of technological progress. Facing the issue of insufficient total demand, expansionary fiscal policy stimulates economic growth, thereby also providing a solid demand foundation and a relaxed macro environment for scientific and technological innovation activities, which helps China to accelerate its catch-up in the field of AI.
In the long term, technological progress and capital deepening also call for changes in the tax system. Digital taxes, robot taxes, and AI taxes have sparked widespread discussions globally in recent years. For China, the direction of increasing direct taxes (especially those related to property) and reducing indirect taxes established at the Third Plenary Session of the 18th Central Committee is a major direction for promoting fairness.
Leave A Comment